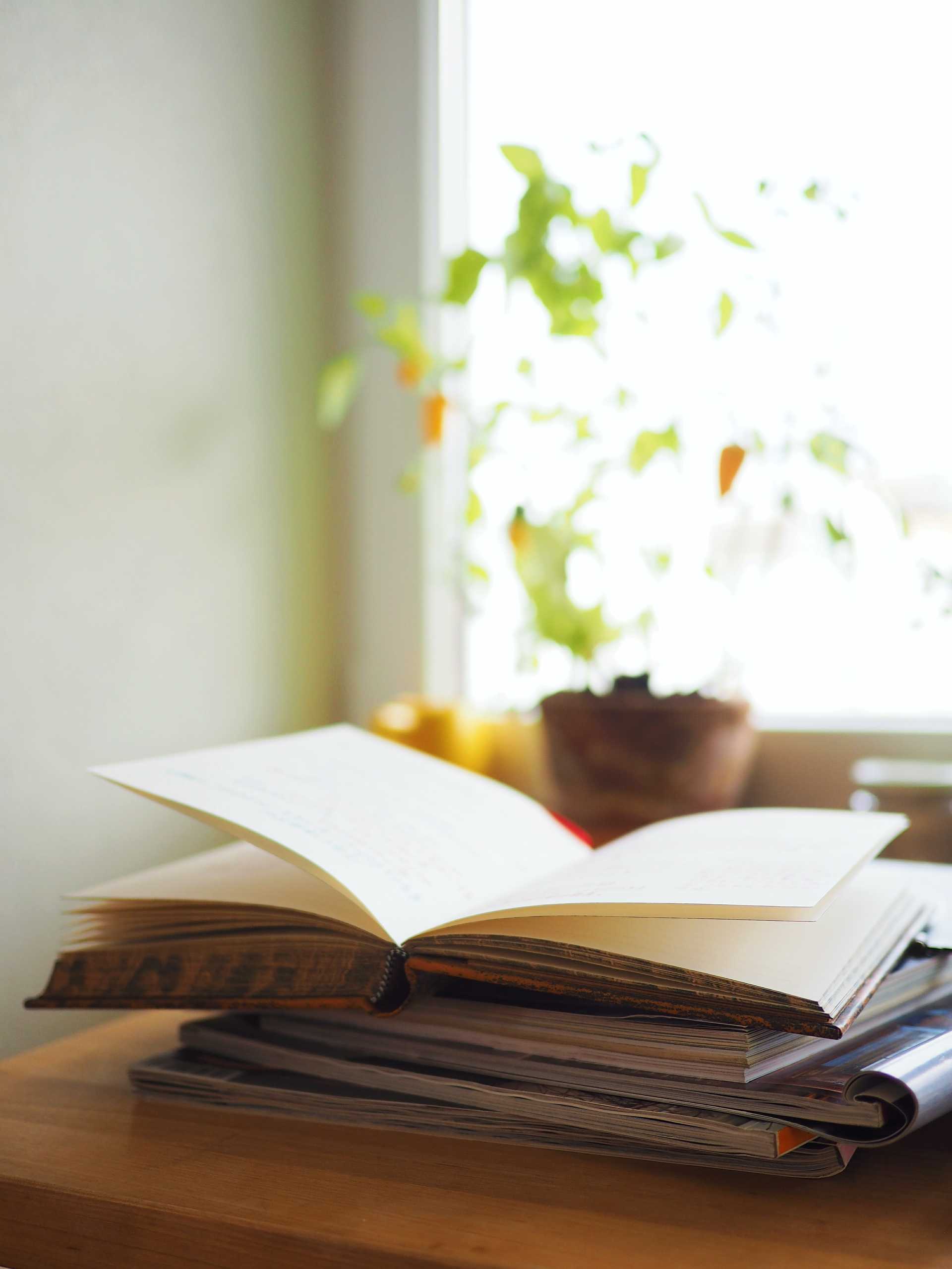
Explainable AI
What and how should an AI system explain itself? A road to collaborative, effective and safe AI systems.
Towards a responsible and effective human–AI collaboration with the help of explainable AI.
Explanations are about communication and is thus bidirectional. There is an entity with a question, human or artifical, and an entity with a potential answer. That answer is rarely ever satisfactory in one go, making explanations a dialogue mechanism for transferring knowledge. These dialogues are initiatied and continue until an information need is satisfied. Explanatory dialogues, when done right, lead to new follow-up questions and will affect future dialogues. Explainable AI is about allowing humans to have explanatory dialogues with AI systems. As a research field, it is about understanding what questions are important and when. Follwed by designing the kind of dialogue that should follow such a question. It is also about giving AI systems the capability of taking part in this dialogue. Not just by offering answers but also interpreting the questions as they are intended. These explanatory dialogues between humans and AI systems are a corner stone of the collaboration between them.
My case for Explainable AI
The potential of artificial intelligence (AI) is ever growing. Our society has begun to experience its benefits and dangers. With this experience also came the realization that we have a limited understanding how our AI systems function. This created for an uneasiness, as many of these systems are designed and engineered to impact our lives in profound ways. This gave rise to the research field of explainable AI with its primary goal of giving us the understanding so we feel we can trust the systems we build for ourselves. We want to understand how, when and why an AI system behaves as it behaves. However, explanations are more then an answer that makes us trust a system. Often a complicated looking piece of information already speaks to the human bias of trusting that what looks complex. It matters suprisingly little if that information makes any sense. As such, explanations need to be carefully designed accounting for the receiving human's context such as their role, expertise, current task and situation. Explanations are also not only useful to calibrate human trust and reliance. They can extend the capabilities of an AI system from a mere tool giving advice or controlling a robot, to a partner making suggestions and participating in delibration processes. An explanation can disclose alternatives and empower humans to take the action - including the action to report an AI system when it is misbehaving or malfunctioning. Explanations also allow more people to interact with AI systems, steering their behaviour or even reprogramming them. Taking this further, explanations can become bidirectional where both human and system learn from each other as they collaborate to complete the task at hand.
PublicationsStudents supervised
Dhivin Nelson
2022
Privacy preserving actionable explanations.
Supervised with Bart Kamphorst (TNO) and Meike Nauta (UT).
Wouter Zirkzee
2021
An exploration of privacy preserving XAI.
Supervised with Mark Neerincx (TuD) and Bart Kamphorst (TNO).
Chantal Leeuwestein
2020
Explainable Artificial Intelligence for decision support systems in financial services.
In collaboration with a financial institute. Supervised with Mark Hoogendoorn (VU).
Manon de Jonge
2020
Simulating team work: Software support for research in human-agent teaming.
Supervised with Frank Grootjens (RU).
Elisabeth Nieuwburg
2019 - Cum Laude
An objective user evaluation of explanations of machine learning based advice in a diabetes context.
Supervised with Mark Neerincx (UU) and Anita Cremers (HU).
PublicationMarcel Robeer
2018 - Cum Laude
Contrastive explanation for machine learning.
Supervised with Matthieu Brinkhuis (UU) and nominated for the 2019 Best Master’s Thesis at Utrecht University.
Publication
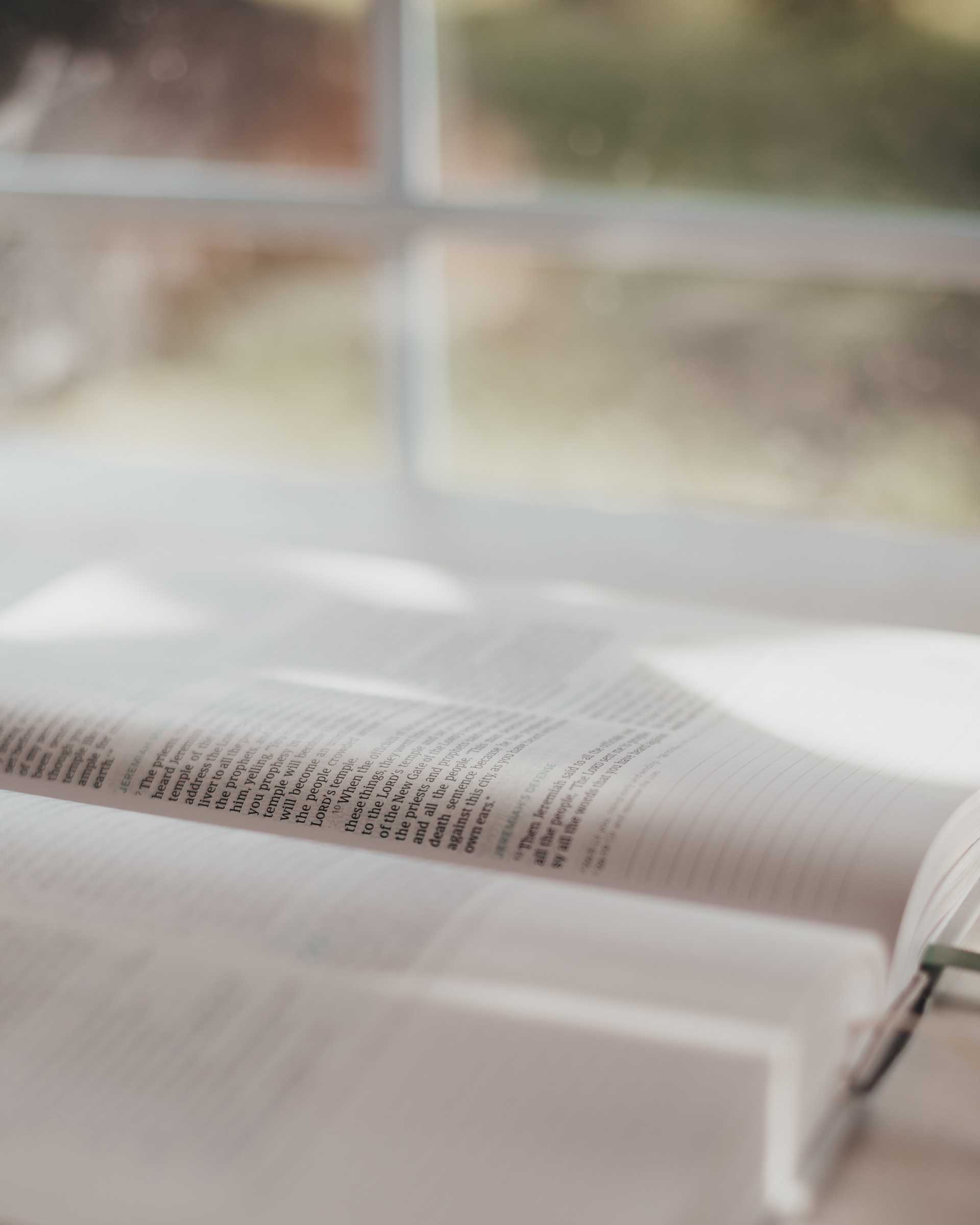