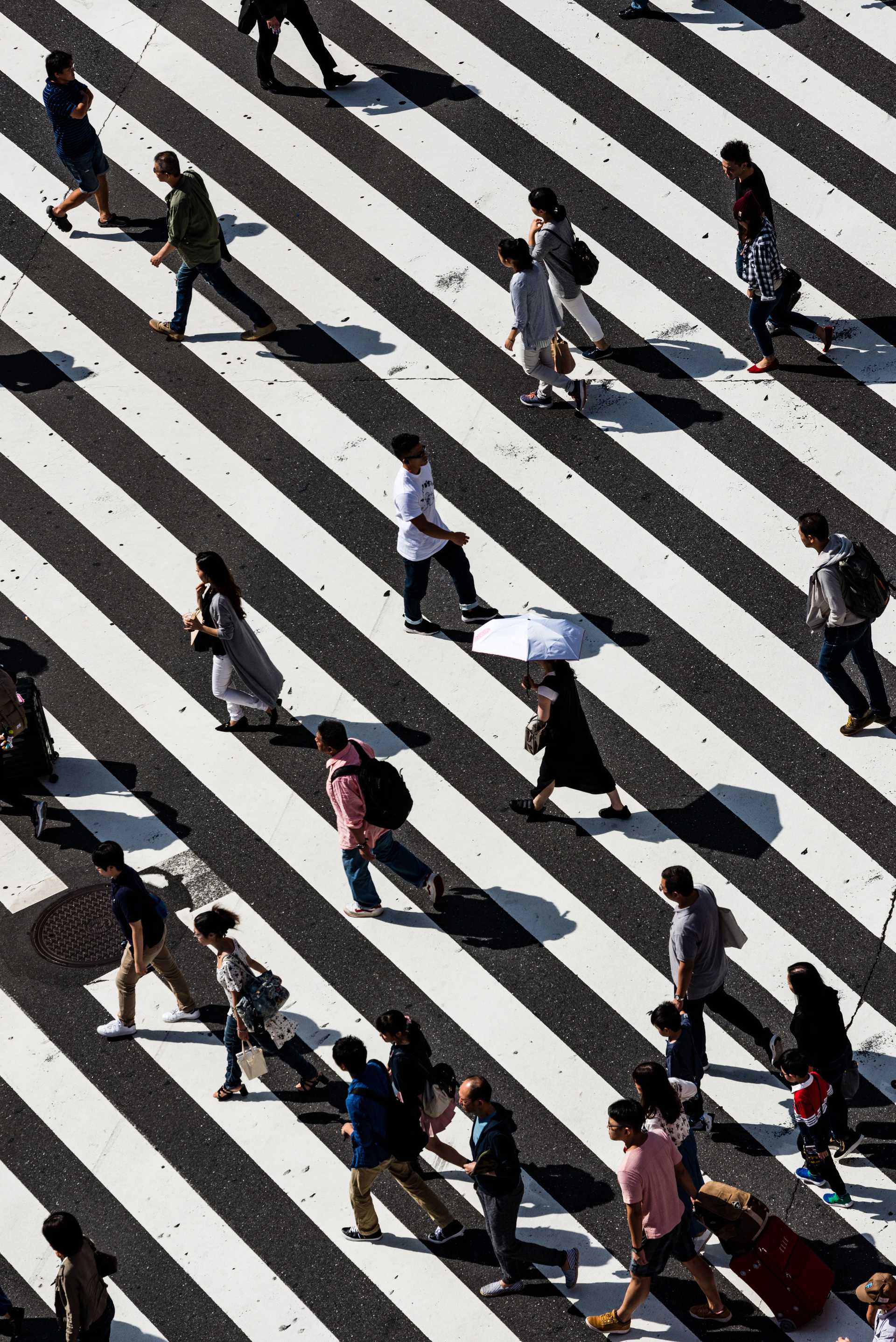
Community and opensource projects
To ensure AI systems are applied and used responsible and effectively, I believe that research and industry communities should to be brought together. I initiate and projects that do so within the organisations I am involved in.
Aside from my Explainable AI research and research at TNO, I am involved in several structural collaborations within projects and organizations such as the Hybrid Intelligence Centre (HI), the Appl.AI Program and the Netherlands AI Centre (NLAIC). I am the founder and maintainer of several open source projects and their communities, and I actively organize community events to bring researchers and industry partners together.
Community events and tools
Building TNO’s XAI community
Increasingly more TNO projects research or make use of explainable AI. I organize or advise in several community events to maintain this community. The goal is to bring this research community into contact with other research and government institutes, relevant groups at universities, and companies through round-table discussions. Through these activities I hope to shape the (applied) research of XAI within the Netherlands towards that what is needed, requested and valued.
H3AI; Hackathon on collaborative fake news detection
I organize the Hackathon on Hybrid Human-AI (H3AI), which is affiliated with TNO’s Appl.AI program that bundles all of TNO’s research on AI and the HHAI Conference linked to the Hybrid Intelligence Centre. The first hackathon tackled fake news detection through the use of hybrid intelligence principles and technologies. The goal is to repeat this hackathon every year during the HHAI conferences.
www.h3ai.nlSummer school Human-Robot Interaction
As part of the European Horizon project TRADR, I helped organize the summer school on human-robot interaction in 2017. During an entire week students were given the opportunity to learn more about this field and network within its community.
HRI Summer schoolHybrid AI Hackathon; Explainable AI
In 2018 I organized my first hackathon with the topic of explainable AI. During this hackathon several teams from various research groups, institutes and companies joined to tackle innovative challenges surround explainable AI. Not only did this result in several new ideas and approaches, it also established several long lasting collaborations on applying explainable AI innovations.
Hackathon videoMATRX: A tool to accelerate research on human-machine teaming
Together with my colleague, Tjalling Haije, we founded the open source tool MATRX and its community. We were confronted time and time again that each project on human-machine teaming required the development of a simplified task environment to test and evaluate human-AI collaborations. Often such testbeds were never used or scalable after the end of a project. With MATRX we solved this as it functions as a singular codebase to accelerate the creation of team tasks, AI and human controlled agents with various (standardized) metrics to enable the benchmarking of research in various projects. Currently MATRX is being used within TNO on key projects, is used by several PhD students in their research, and found its place in the assignments of various courses at the Technical University of Delft.
www.matrx-software.comXAI Toolbox: Standardized plug-and-play technologies for explainable AI
My most recent open source project that aims to collect and standardize much of the explainable AI (XAI) research done within TNO and outside in a single Python open source package. Its goal is to consolidate the technology oriented research on XAI research in a single accessible way to help support the growing research community on this topic and to accelerate the application of this research.
Current TNO projects
Hybrid Intelligence in Practice
Hybrid Intelligence encompasses the idea that the next generation of AI systems, are systems that collaborate in symbioses with humans. This project, of which I am the project leader, has as goal to evaluate the readiness of Hybrid Intelligence in societal relevant use cases. Within this project we do so by attempting to build one of the first Hybrid Intelligence applications within the healthcare domain.
Responsible XAI for the military
The responsible application of explainable AI is not apparant as we only know little on how to communicate explanations and what their effects are. I am leading a project on how XAI can and should be applied responsibly in high-risk AI applications for the Dutch military.
Explainable AI for decision support
The research line, led by myself, within TNO’s Appl.AI program on the requirements, interaction designs and technologies needed for an AI system to explain itself to various human stakeholders. Several AI use cases are tackled to explore and generalize the usefulness of explainable AI.
Appl.AIExplainable AI in smart energy systems
A project in which I advise on the potential of Explainable AI research in AI applications for consumers to manage their energy usage at home and energy suppliers to optimize the energy grid for households.
AI safety and artificial Moral models
A project to develop a methodology to design artificial moral models in an iterative fashion to enable responsible high-risk applications of AI. In my role as scientific advisor, I aid the team in developing this methodology that utilizes state of the art human-AI interaction technologies such as conversational AI and explainable AI.
Delegation in human-AI teams
Research on how humans can effectively and responsibly delegate autonomous systems and virtual agents in an efficient way that leverages the intelligences of these systems and agents as well as the unique human capabilities. Under my lead we develop the DASH concept.
DASH
Past projects
Research roadmap on the operationalization of AI and data science
Together with other researchers from various domains this projects works towards a roadmap how to operationalize AI and data science. My role was to advise and provide a vision towards the topic of human-machine teaming and how it should be operationalized. I led a team of various researchers and practitioners of AI from different research institutes and companies.
Intelligent operator support system
Within the European Horizon project MOSES, I led the research on how AI-based support tools can be developed to support operators in supervising not one, but many autonomous operations in parallel in a safe and effective manner.
MOSESResearch roadmap on Human-Machine Teaming
A project to establish the second iteration of the roadmap on human-machine teaming research for the Dutch Department of Defense (DoD). Within this roadmap we defined the research areas DoD and their investment priorities to ensure their use of AI and autonomy is done effectively and in a responsible manner.
Actionable explanations through causal models
Those who are subjected to an AI system’s decision need the ability to contest those decisions. This research resulted in a method that combines machine learning and causal models to generate explanations that support this ability by explaining what steps the subject can take to influence the AI system’s decision (e.g., change their lifestyle( or report their concerns effectively (e.g., report a biased decision or a violation of privacy).
Operator support on maritime vessels
Current large maritime vessels have a sophisticated auto-pilot to follow a set course or to manage a position, even under extreme weather conditions. Within the project we developed a concept that allows a single operator to supervise this auto-pilot while roaming the vessel. This is made possible through predictive analytics such that the operator can be informed in time to return to the bridge to manage issues and deviations.
Operator support videoMachine learning on EEG data for an improved virtual reality experience
Latency is an issue for any virtual reality experience, where head movements are out of sync of what is projected. With a patented approach of EEG data and machine learning we managed to predict up to 0.5 seconds in the future if someone will move its head and in which direction.